Microsoft Lobeご存知でしょうか?
画像分類を行う機械学習モデルを無料かつノーコードで作成できるアプリケーションです。
最近のMicrosoftは、Microsoft Clarityも無料で提供してくれているので私の中で評価あがっています。
会議の自動文字起こしアプリなんかも出しています。これが超絶便利で、リアルタイムで文字起こしをしてくれます。
機械学習とかは興味があるけど、難しそうでなかなか手を出しづらかったりします。
画像分類のアプリを作成するときの流れは、以下のようになります。
- 学習用画像データを収集し極力ノイズがないようにする。
- 上記の画像を利用して学習モデルを生成する。
- 学習したモデルを利用して、アプリを作成する。
Lobeでは、上記の手順のうち、2の部分と3のアプリで利用する形式での学習モデルのエクスポートまでを行ってくれます。
Tensorflowのサンプルコード(retrain.py)で以前に機械学習で写真分類のモデルを作成したことがありますが
Lobeを使うと、直感的に、簡単に分類モデルを作成することができます。
今回はネタとして、マッチョ度判定をするWebアプリ作成までの手順をお届けします。
画像を選択すると、以下の体型類推を行うものです。
- ボディービルダー
- フィジーク
- ベストボディー
- ガリガリ
- ガリガリ君
- お相撲さん
2のモデル作成は簡単にできたのですが、3のWebアプリへの落とし込みの部分が、環境が合わなかったりサンプルコードが少なく色々と試行錯誤しました。そちらについても、合わせてご紹介したいと思います。
試行錯誤した挙げ句、結局ブラウザ側で、TensorFlow.jsを利用して類推する実装になりました。
Lobeで、学習したモデルのExport形式は、以下の通りです。
- CoreML
- TensorFlow Lite
- TensorFlow.js
- TensorFlow
- ONNX
- Local API
サーバ側で実行したり、ブラウザ側で実行することもできます。
まずはじめに、肝となる学習データの集め方について記載します。
目次
学習用の画像収集
機械学習では、この学習データ(教師データ)が非常に重要になります。
教師データの中に全く違った画像が混じってしまうと予測の精度が落ちてしまいます。
ある程度一定のクォリティーの画像を大量に集める必要があります。
学習用のデータを一つずつ集めるのは大変なので、Googleが提供しているツール(google-image-downloader)を利用します。
前提として以下が必要になります。
- python3
- pip
こちらは、ローカルのMacで実行するものなので、Macに入れておきます。
# brew install python
# echo 'export PATH="/usr/local/opt/python@3.8/bin:$PATH"' >> /Users/xxxxxx/.bash_profile
上記で、pipも同時にインストールされます。
以下のコマンドで、google_images_downloadをインストールするのですが、実行しても画像のダウンロードが始まりません。
# pip install google_images_download
# googleimagesdownload -k "test"
Item no.: 1 --> Item name = test
Evaluating...
Starting Download...
Unfortunately all 100 could not be downloaded because some images were not downloadable. 0 is all we got for this search filter!
Errors: 0
Everything downloaded!
Total errors: 0
Total time taken: 0.9399161338806152 Seconds
こちらは、パッチがあたったものが公開されているので、そちらをgit cloneして、直接コマンド実行していきます。
# git clone https://github.com/Joeclinton1/google-images-download.git gid-joeclinton
# python3 gid-joeclinton/google_images_download/google_images_download.py -k "test" --limit 10
Item no.: 1 --> Item name = test
Evaluating...
Starting Download...
URLError on an image...trying next one... Error: HTTP Error 462:
Completed Image ====> 1.general-test.jpg
Completed Image ====> 2.road-sign-361513_960_720.jpg
Completed Image ====> 3.risky-assumptions.jpg
Completed Image ====> 4.test%20100%25-300x0.png
Completed Image ====> 5.logo%20test%20horiz%20bis.png
Completed Image ====> 6.the-test-fun-for-friends-screenshot.jpg.png
Completed Image ====> 7.783px-test-logo.svg.png
Completed Image ====> 8.conducting-usability-test_yon4bqt.svg
Completed Image ====> 9.standardized%20test.jpg
Completed Image ====> 10.test_sp.jpg
Errors: 1
Everything downloaded!
Total errors: 1
Total time taken: 12.184973001480103 Seconds
上記の実行により、ディレクトリのdownloadsディレクトリ内に、キーワード毎の画像がダウンロードされます。
オプション | 内容 |
---|---|
-k | 取得したい画像のキーワード 例) リンゴ |
-l | 画像の取得数 例) 100 |
-f | ダウンロードしたい画像のフォーマット 例) jpg, gif, png |
-nn | 画像に数字を振らない |
-s | 画像のサイズ 例) large, medium, icon, >400×300, >640×480, >800×600, >1024×768, >2MP, >4MP, >6MP, >8MP, >10MP |
実際に、今回使用するボディービルダーの画像を収集してみます。
# python3 gid-joeclinton/google_images_download/google_images_download.py -k 'ボディビルダー' -l 10 -f jpg -nn -s medium
画像を確認してみます。downloadsディレクトリに、ボディービルダーディレクトリができ、画像が格納されています。
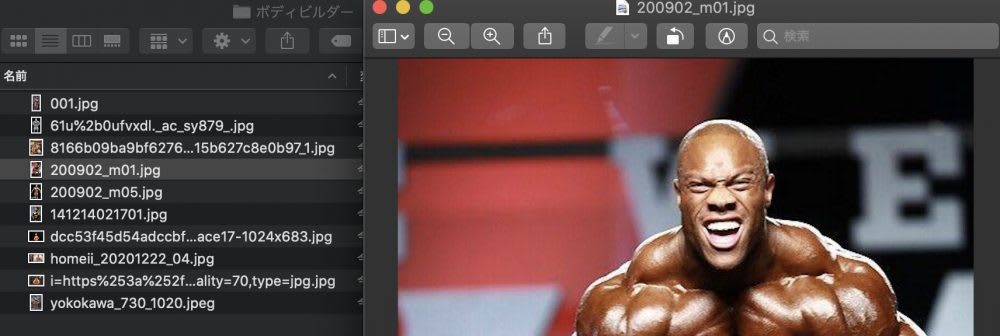
このようにして、機械学習の学習の為のデータを集めていきます。
目視で確認して、あまりにも学習データとしてふさわしくないものはこの時点で削除しておきましょう。
また、学習データとしては、1つのカテゴリで、100以上の画像は必要かと思います。この数が多ければ多いほど精度はあがります。
Microsoft Lobeで学習モデル生成
ダウンロード&インストール
まずは、Microsoft Lobeを以下からダウンロード、インストールします。
2021/02/26(金)時点では、Beta版です。Mac版、Windows版があります。
名前とメールアドレスを入力してDownloadボタンを押すとダウンロードが始まります。
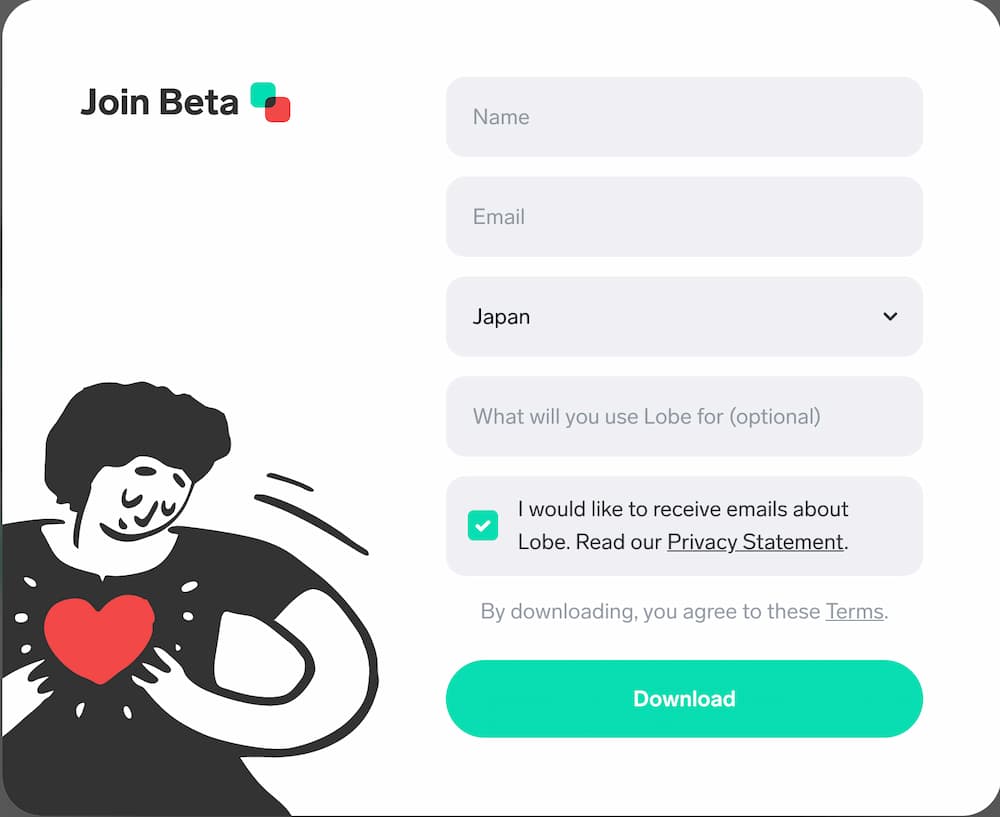
zip形式でダウンロードされるので、解凍すると、Lobeというアプリができます。
アプリケーションディレクトリに移動しておくとよいでしょう。

Lobe起動とプロジェクト生成
起動してみます。
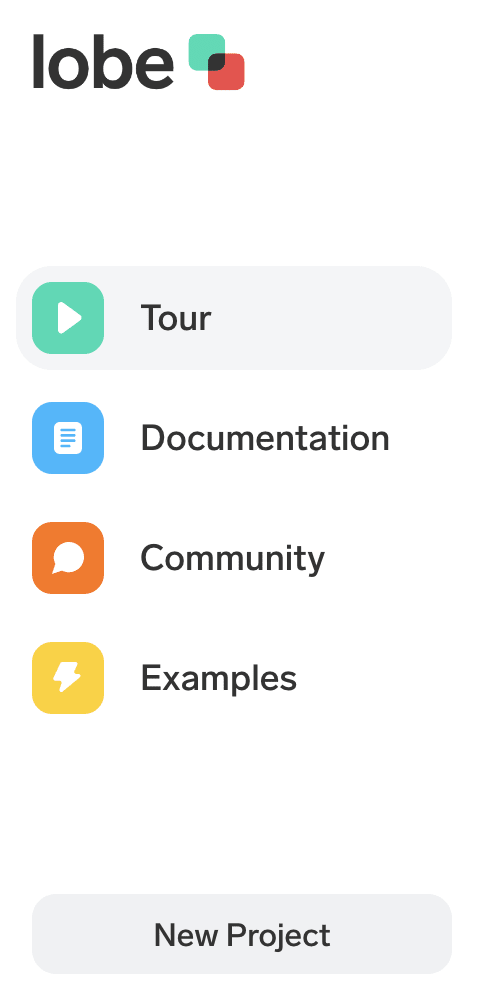
New Projectボタンから、プロジェクトを新規作成します。
プロジェクトのタイトルですが、モデルをエクスポートする際にエラーが発生することがあったので、できれば英語でつけたほうが良さそうです。
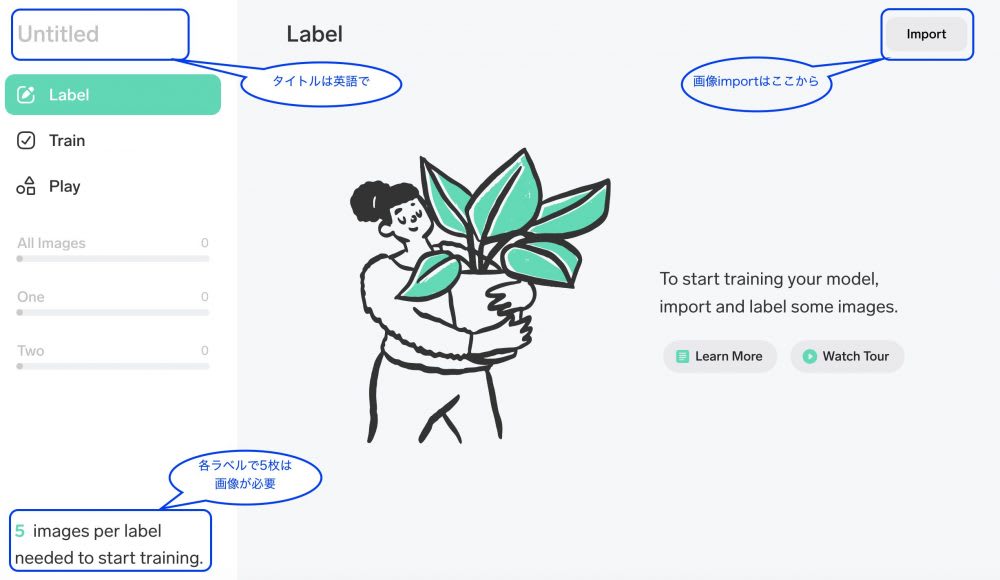
画像の取り込み
事前に、収集した画像をLobe上に取り込んでいきます。
画像の取り込み方法は、Images、Camera、Datasetの3種類で、今回はDatasetで取り込んで行きます。
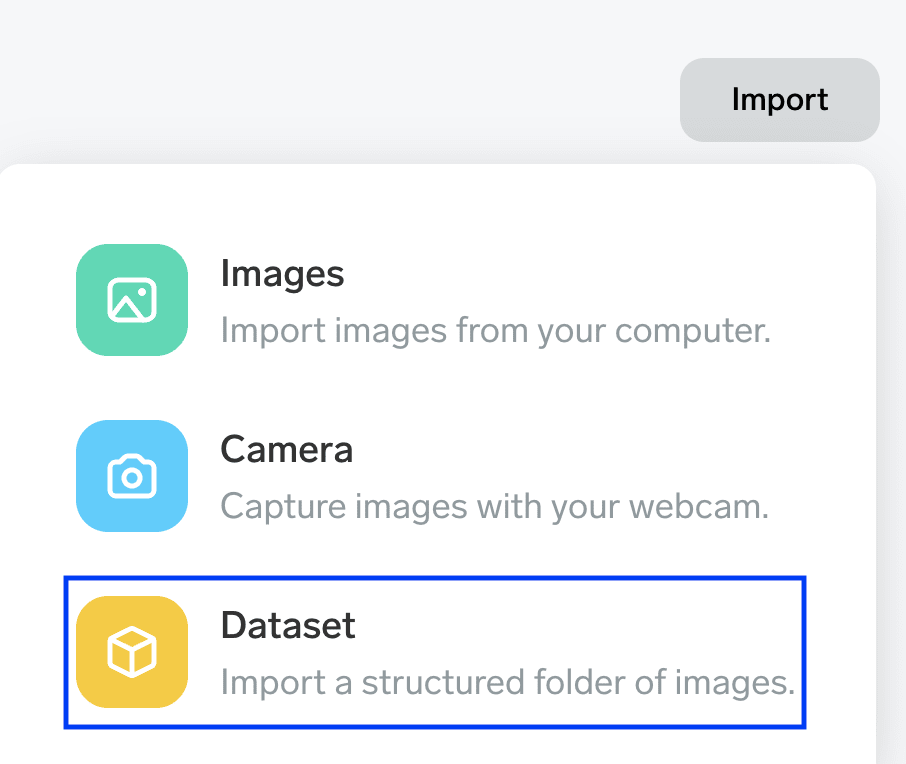
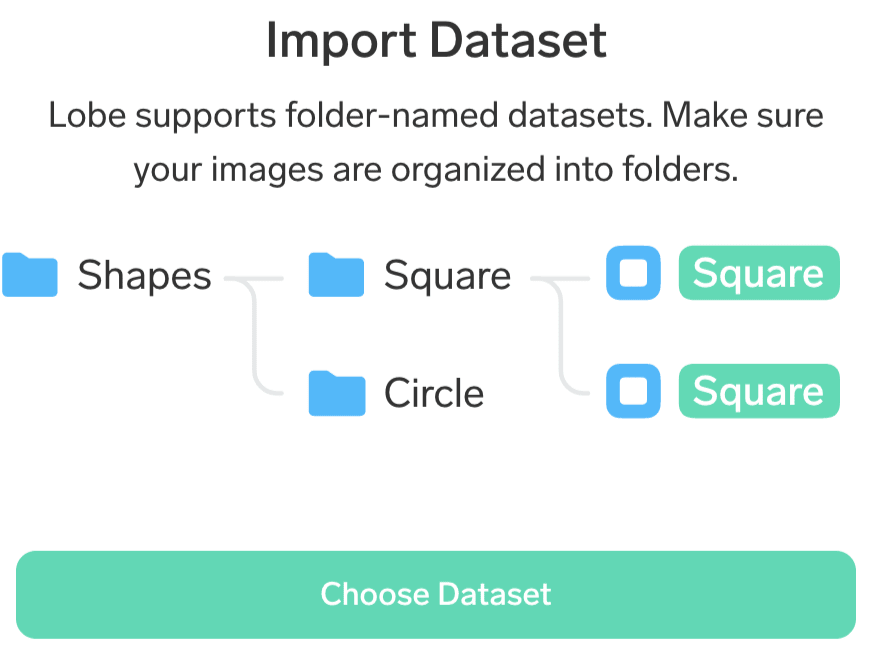
ファイル選択画面が出るので、google images downloadで集めた画像ディレクトリを指定します。
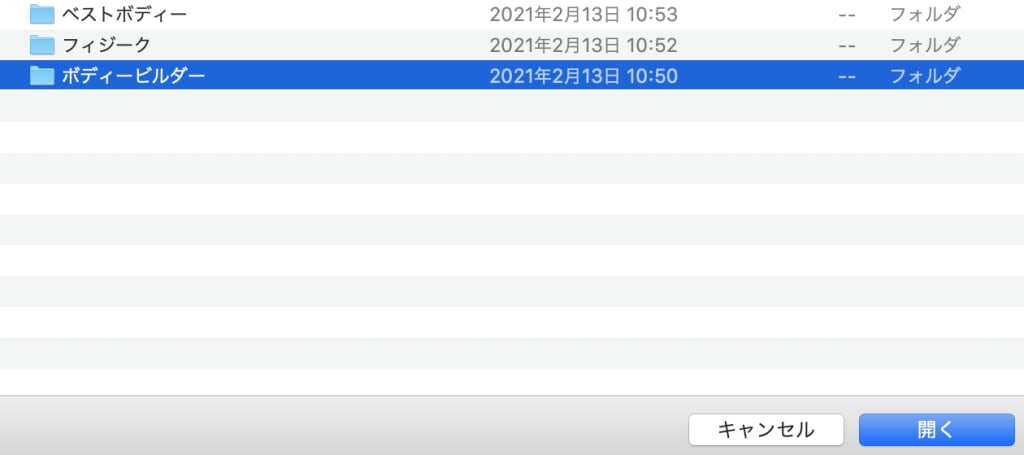
ラベルは、どうするんだいって聞かれるので、フォルダー名を利用するを選択してインポートします。
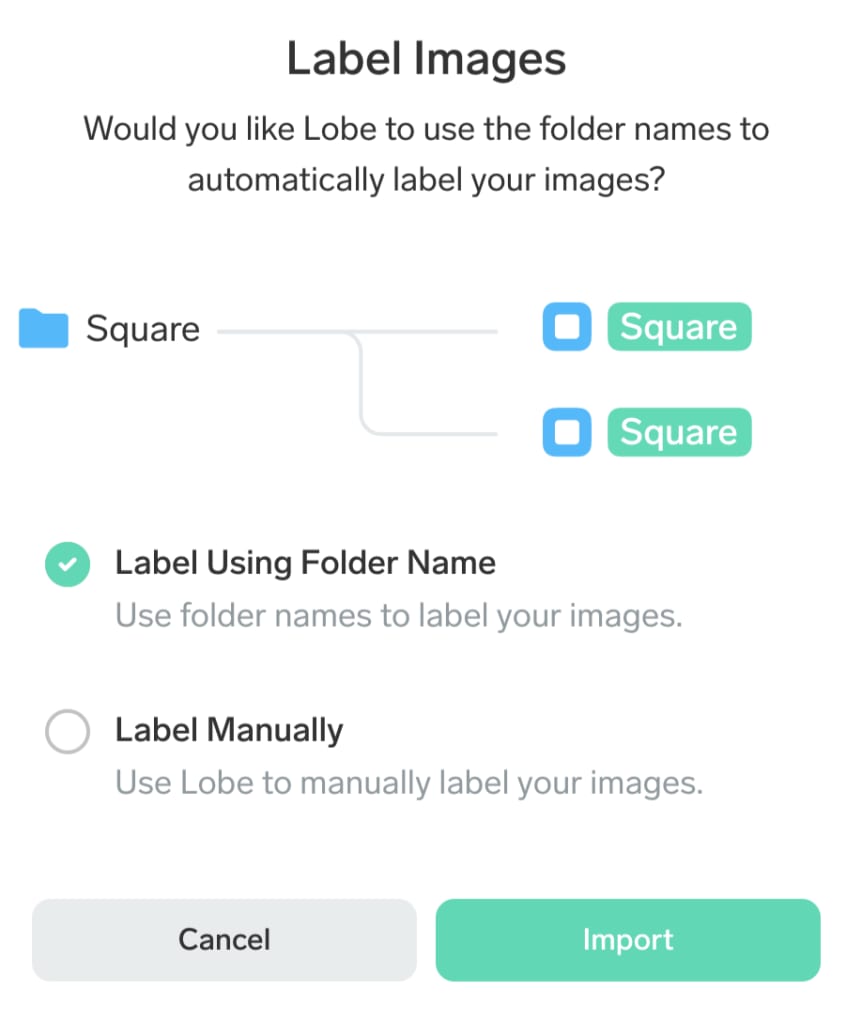
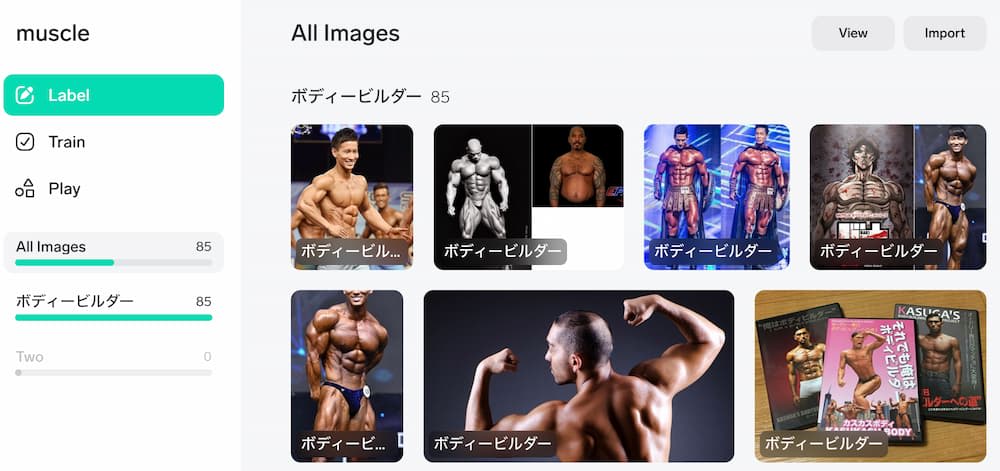
同様にして、分類したい画像をすべて取り込んでいきます。
今回の分類は、お相撲さん、ガリガリ、ガリガリ君、フィジーク、ベストボディー、ボディービルダーの6つに分類するので、全て取り込みます。
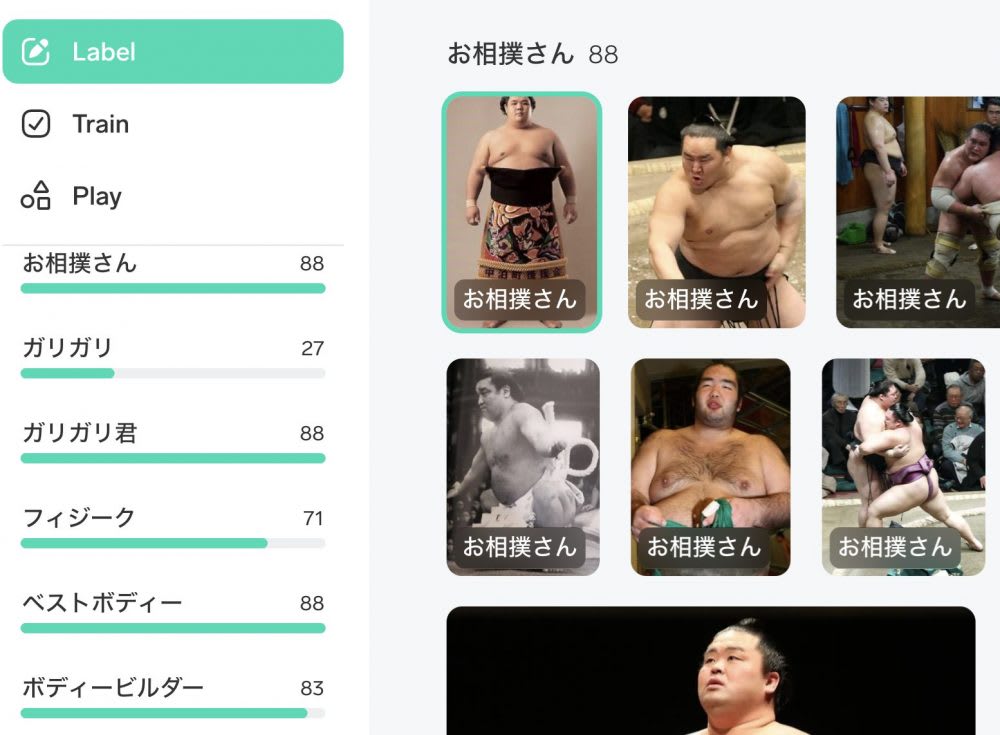
Lobeによる学習
前項で、分類したい画像がすべて取り込めたら、Trainボタンを押して、学習させます。
写真の数、ラベルの多さにより、時間がかかります。
以下のように学習した結果が表示されます。
精度をあげるには、写真の数を多くして、誤った写真が紛れ込まないようにすることが大事です。
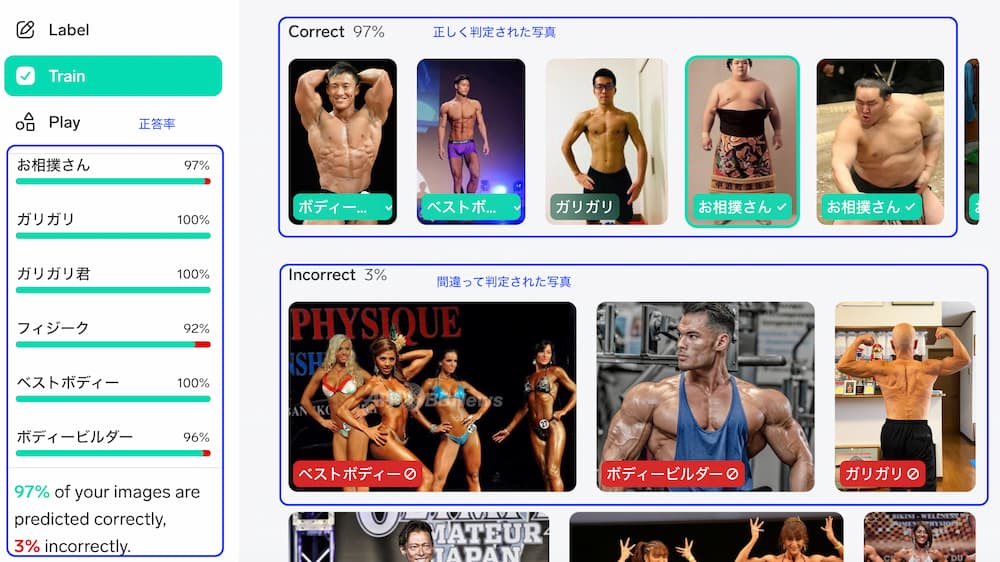
Labelから、取り込んだ画像を見れるので、判定が難しい画像等は、削除して、他の画像を追加して精度をあげましょう。
Lobeによる予測確認
左ナビから、Playを選択して、判定する画像をdrag & dropで置きます。
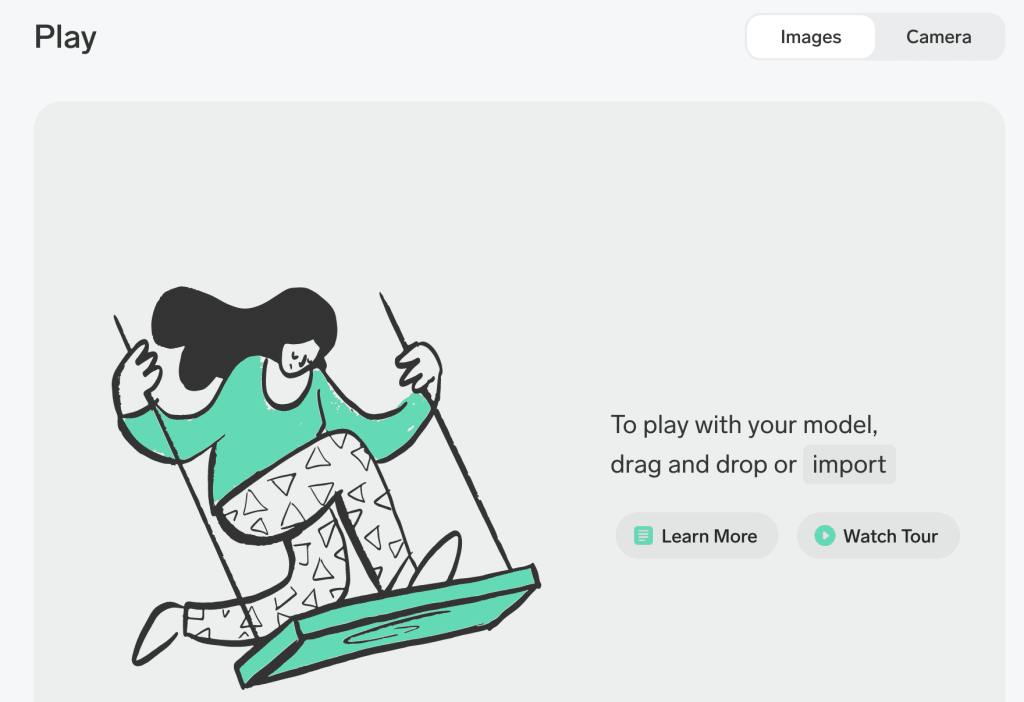
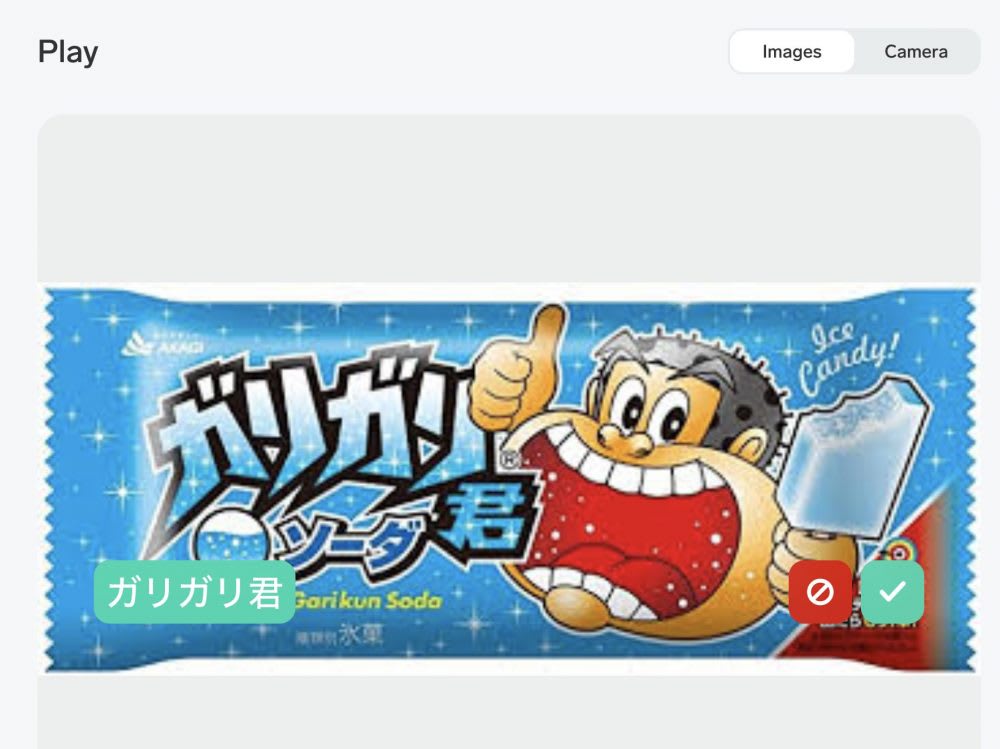
チェックマークを押すと、学習用のデータとして取り込まれ、さらにモデルの精度が上がっていきます。
モデルのエクスポート
実際に、この学習したモデルを使ってアプリを作る場合は、エクスポートする必要があります。
最初は、phpのファイルアップロード処理時に、外部コマンドとして、TensorFlowを実行して写真の分類を行おうと考えました。
TensorFlow形式(おそらくver2形式)で出力しましたが、実行しようとしたサーバが、AVX(Advanced Vector Extensions)をサポートしていないCPUの為、TensorFlow1.5しか動かず、うまくコマンド実行できませんでした。
そのため、最終的には、TensorFlow.js形式で出力しました。
プロジェクト名を日本語にしている場合、TensorFlow.jsのエクスポートでエラーが発生したのでご注意ください。ファイルメニューから、Exportを選択します。
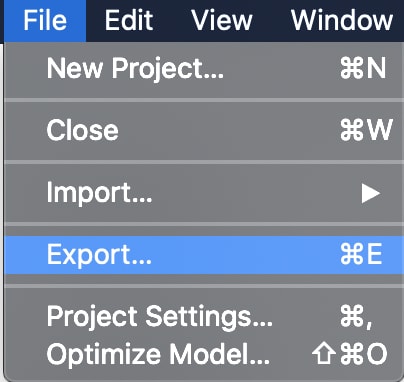
TensorFlow.jsを選択し、出力先ディレクトリを指定します。
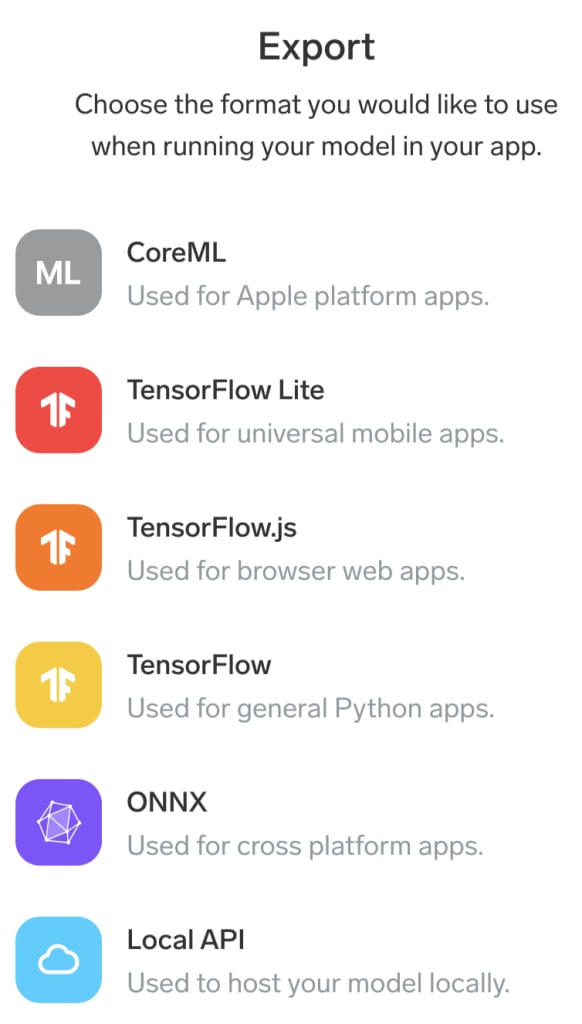
最適化するかどうか聞かれるので、Optimize & Exportを選択して、Exportします。
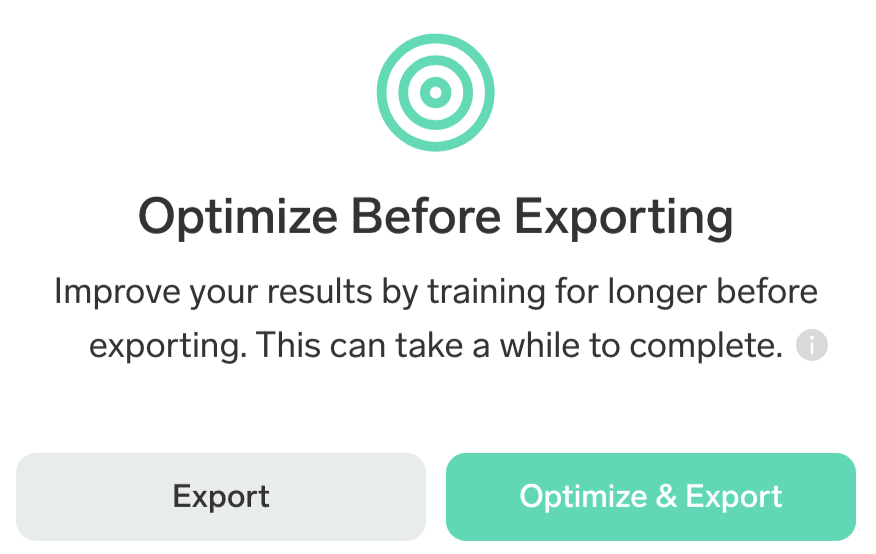
以下のようなファイル群が出力されます。
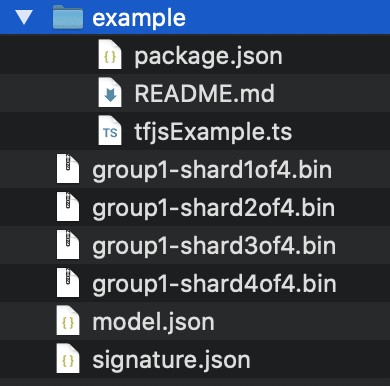
アプリの実装
最終的にうまくいった方法を紹介します。
前項で、出力した、TensorFlow.js形式のデータを利用しました。
モデルのディレクトリをサーバ上の公開ディレクトリに設置します。
automlを利用するため、↑のディレクトリに、dict.txtというラベルの文字列を羅列したものを設置します。
# cat dict.txt
お相撲さん
ガリガリ
ガリガリ君
フィジーク
ベストボディー
ボディービルダー
上記のファイルの並び順は、Lobeの左ナビの順番になります。
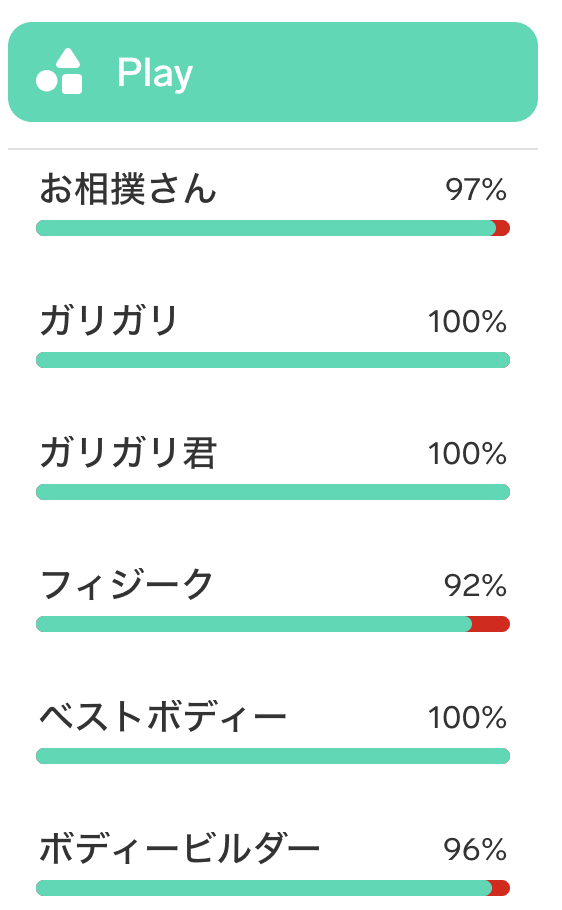
コードとしては、以下になります。
以下のscriptを読み込みます。
<script src="https://unpkg.com/@tensorflow/tfjs"></script>
<script src="https://unpkg.com/@tensorflow/tfjs-automl"></script>
以下でモデルをロードします。
let model;
async function init() {
model = await tf.automl.loadImageClassification('https://zaitakukinmu.com/models/model.json');
}
以下で、分類をします。
async function predict() {
let image = document.getElementById('previewImage');
const predictions = await model.classify(image);
console.log(predictions);
}
predictionsの中身は以下のような中身になります。
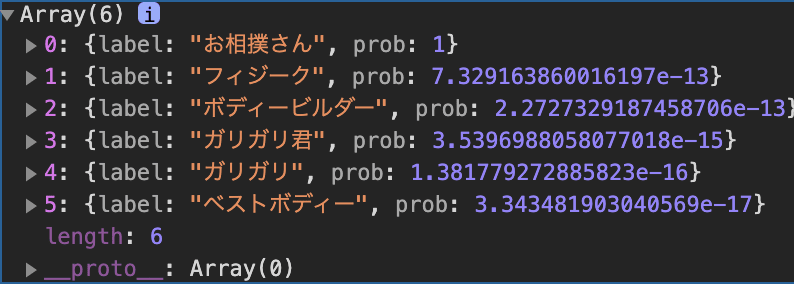
出来上がり
試行錯誤色々
- まずは、TensorFlow形式でサーバ側で実行しようとしたが、TensorFlowは2系にする必要があるが、当サーバが、AVXに対応しておらず、仕方なく、TensorFlow1.5にするが、モデルの形式が合わずエラー。
- それならと、サーバサイド(node)で、TensorFlow.jsをAPIで実行しようとしてみたが、TensorFlowと同じように、Illegal instruction(Core Dump)が発生して実行できず。(AVXに対応していないため)
上記から、そもそもサーバを変えるぐらいしか打つ手がなさそうなので、一旦は、Webブラウザでの実行形式にしました。
[失敗したやつ]TensorFlow.js形式で、サーバ側で実行
TensorFlow形式でエクスポートしたディレクトリに中に、exampleディレクトリがあります。
ここに、サンプルコードがあるので、これを実行していきます。
package.jsonがあるので、npm installで必要なnode moduleをインストールします。
# npm install
npm WARN deprecated node-pre-gyp@0.14.0: Please upgrade to @mapbox/node-pre-gyp: the non-scoped node-pre-gyp package is deprecated and only the @mapbox scoped package will recieve updates in the future
> @tensorflow/tfjs-node@2.8.6 install /var/www/zaitakukinmu/models/example/node_modules/@tensorflow/tfjs-node
> node scripts/install.js
/var/www/zaitakukinmu/models/example/node_modules/@tensorflow/tfjs-node/scripts/install.js:109
async function ensureDir(dirPath) {
^^^^^^^^
SyntaxError: Unexpected token function
at createScript (vm.js:56:10)
at Object.runInThisContext (vm.js:97:10)
at Module._compile (module.js:549:28)
at Object.Module._extensions..js (module.js:586:10)
at Module.load (module.js:494:32)
at tryModuleLoad (module.js:453:12)
at Function.Module._load (module.js:445:3)
at Module.runMain (module.js:611:10)
at run (bootstrap_node.js:394:7)
at startup (bootstrap_node.js:160:9)
LobeTensorFlowJSExample@1.0.0 /var/www/zaitakukinmu/models/example
├── @types/node@14.14.31
├─┬ ts-node@9.1.1
│ ├── arg@4.1.3
│ ├── create-require@1.1.1
│ ├── diff@4.0.2
│ ├── make-error@1.3.6
│ ├─┬ source-map-support@0.5.19
│ │ ├── buffer-from@1.1.1
│ │ └── source-map@0.6.1
│ └── yn@3.1.1
└── typescript@4.1.5
npm WARN LobeTensorFlowJSExample@1.0.0 No repository field.
npm ERR! Linux 3.10.0-514.26.2.el7.x86_64
npm ERR! argv "/usr/bin/node" "/bin/npm" "install"
npm ERR! node v6.17.1
npm ERR! npm v3.10.10
npm ERR! code ELIFECYCLE
npm ERR! @tensorflow/tfjs-node@2.8.6 install: `node scripts/install.js`
npm ERR! Exit status 1
npm ERR!
npm ERR! Failed at the @tensorflow/tfjs-node@2.8.6 install script 'node scripts/install.js'.
npm ERR! Make sure you have the latest version of node.js and npm installed.
npm ERR! If you do, this is most likely a problem with the @tensorflow/tfjs-node package,
npm ERR! not with npm itself.
npm ERR! Tell the author that this fails on your system:
npm ERR! node scripts/install.js
npm ERR! You can get information on how to open an issue for this project with:
npm ERR! npm bugs @tensorflow/tfjs-node
npm ERR! Or if that isn't available, you can get their info via:
npm ERR! npm owner ls @tensorflow/tfjs-node
npm ERR! There is likely additional logging output above.
npm ERR! Please include the following file with any support request:
npm ERR! /var/www/zaitakukinmu/models/example/npm-debug.log
エラーになりました。どうも、nodeのバージョンが古いことによるエラーです。
node, npmの環境を整えていきます。
バージョンが古かったので、一旦削除します。
yum remove nodejs npm
nodeのレポジトリをインストールします。
# yum install https://rpm.nodesource.com/pub_12.x/el/7/x86_64/nodesource-release-el7-1.noarch.rpm
yum infoでバージョン12が表示されることを確認します。
# yum info nodejs
利用可能なパッケージ
名前 : nodejs
アーキテクチャー : x86_64
エポック : 2
バージョン : 12.20.2
リリース : 1nodesource
容量 : 22 M
リポジトリー : nodesource/x86_64
要約 : JavaScript runtime
URL : http://nodejs.org
ライセンス : MIT and ASL 2.0 and ISC and BSD
説明 : Node.js is a platform built on Chrome's JavaScript runtime
: for easily building fast, scalable network applications.
: Node.js uses an event-driven, non-blocking I/O model that
: makes it lightweight and efficient, perfect for data-intensive
: real-time applications that run across distributed devices.
nodeをインストールします。
# yum install nodejs
# node --version
v12.20.2
# npm --version
6.14.11
再度、npm installをします。
# npm install
成功しました。
exampleディレクトリで、以下のコマンドを実行します。
npm run predict path/to/your/image
以下のエラーが発生しました。
# npm run predict ../../72632125_163x291.jpeg
> LobeTensorFlowJSExample@1.0.0 predict /var/www/zaitakukinmu/models/example
> ts-node tfjsExample.ts "../../72632125_163x291.jpeg"
/var/www/zaitakukinmu/models/example/node_modules/@tensorflow/tfjs-node/dist/index.js:49
throw new Error("The Node.js native addon module (tfjs_binding.node) can not " +
^
Error: The Node.js native addon module (tfjs_binding.node) can not be found at path: /var/www/zaitakukinmu/models/example/node_modules/@tensorflow/tfjs-node/lib/napi-v7/tfjs_binding.node.
Please run command 'npm rebuild @tensorflow/tfjs-node build-addon-from-source' to rebuild the native addon module.
If you have problem with building the addon module, please check https://github.com/tensorflow/tfjs/blob/master/tfjs-node/WINDOWS_TROUBLESHOOTING.md or file an issue.
at Object.<anonymous> (/var/www/zaitakukinmu/models/example/node_modules/@tensorflow/tfjs-node/dist/index.js:49:11)
at Module._compile (internal/modules/cjs/loader.js:999:30)
at Object.Module._extensions..js (internal/modules/cjs/loader.js:1027:10)
at Module.load (internal/modules/cjs/loader.js:863:32)
at Function.Module._load (internal/modules/cjs/loader.js:708:14)
at Module.require (internal/modules/cjs/loader.js:887:19)
at require (internal/modules/cjs/helpers.js:74:18)
at Object.<anonymous> (/var/www/zaitakukinmu/models/example/tfjsExample.ts:6:1)
at Module._compile (internal/modules/cjs/loader.js:999:30)
at Module.m._compile (/var/www/zaitakukinmu/models/example/node_modules/ts-node/src/index.ts:1056:23)
npm ERR! code ELIFECYCLE
npm ERR! errno 1
npm ERR! LobeTensorFlowJSExample@1.0.0 predict: `ts-node tfjsExample.ts "../../72632125_163x291.jpeg"`
npm ERR! Exit status 1
npm ERR!
npm ERR! Failed at the LobeTensorFlowJSExample@1.0.0 predict script.
npm ERR! This is probably not a problem with npm. There is likely additional logging output above.
npm ERR! A complete log of this run can be found in:
npm ERR! /root/.npm/_logs/2021-02-20T21_28_55_012Z-debug.log
上記のメッセージに、以下のコマンド実行をするように書いてあるので、以下を実行。こちらもエラー。
# npm rebuild @tensorflow/tfjs-node build-addon-from-source
> @tensorflow/tfjs-node@2.8.6 install /var/www/zaitakukinmu/models/example/node_modules/@tensorflow/tfjs-node
> node scripts/install.js
CPU-linux-2.8.6.tar.gz
(node:11869) UnhandledPromiseRejectionWarning: Error: EACCES: permission denied, mkdir '/var/www/zaitakukinmu/models/example/node_modules/@tensorflow/tfjs-node/deps'
(node:11869) UnhandledPromiseRejectionWarning: Unhandled promise rejection. This error originated either by throwing inside of an async function without a catch block, or by rejecting a promise which was not handled with .catch(). To terminate the node process on unhandled promise rejection, use the CLI flag `--unhandled-rejections=strict` (see https://nodejs.org/api/cli.html#cli_unhandled_rejections_mode). (rejection id: 1)
(node:11869) [DEP0018] DeprecationWarning: Unhandled promise rejections are deprecated. In the future, promise rejections that are not handled will terminate the Node.js process with a non-zero exit code.
@tensorflow/tfjs-node@2.8.6 /var/www/zaitakukinmu/models/example/node_modules/@tensorflow/tfjs-node
エラーが解消されないので、nodeのバージョンを12から14に変えます。
# yum install https://rpm.nodesource.com/pub_14.x/el/7/x86_64/nodesource-release-el7-1.noarch.rpm
以下で入れ直そうとすると、pub_14.xにしたのに、なぜかそのパスで12のものを取得しようとしてエラーになったので、直接rpmをインストール。
# yum install nodejs
rpm -Uvh https://rpm.nodesource.com/pub_14.x/el/7/x86_64/nodejs-devel-14.15.5-1nodesource.x86_64.rpm
インストールが成功したので、npmもアップグレードします。
# node --version
v14.15.5
# npm --version
6.14.11
# npm install -g npm
7.5.4
上記の状態にして、一旦、node_modulesディレクトリを削除して、再度npm installを実施。
# rm -fr node_modules
# npm install
そして以下のコマンドを実行。エラーもでることなく完了。
# npm rebuild @tensorflow/tfjs-node build-addon-from-source
rebuilt dependencies successfully
そして、画像の分類コマンドを再度実行します。
# npm run predict ../../72632125_163x291.jpeg
> LobeTensorFlowJSExample@1.0.0 predict
> ts-node tfjsExample.ts "../../72632125_163x291.jpeg"
Illegal instruction
↑これは、AVXに対応していないCPUの為のエラー。。。。
サーバ変更を検討しないとな。。。。